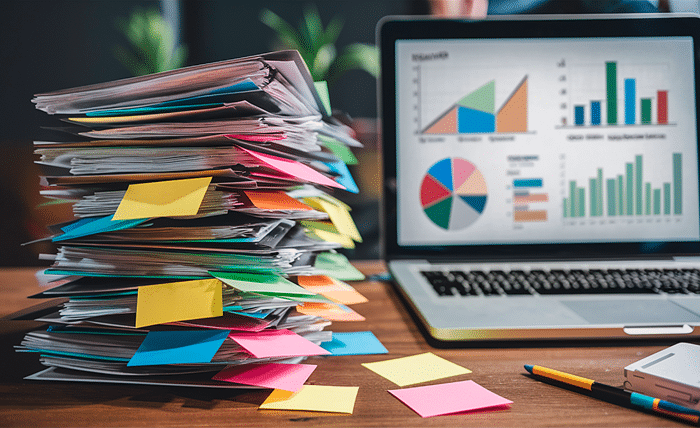
In the rapidly evolving landscape of artificial intelligence (AI) and machine learning (ML), the efficiency of data processing methods has become a paramount concern for businesses and researchers alike. One of the most critical, yet resource-intensive, stages in developing machine learning models is data labeling. This is where active learning, a semi-supervised machine learning algorithm, steps in as a game-changer, promising to streamline data labeling and significantly reduce costs.
Understanding Active Learning
Active learning is a form of machine learning where the algorithm selectively queries the user (or some other information source) to label data points with the greatest expected benefit for the learning process. Unlike traditional methods that attempt to label vast datasets entirely, active learning focuses on the most informative data, thereby reducing the workload and improving model accuracy.
For instance, in image recognition tasks, instead of manually labeling millions of images, active learning algorithms can identify which images are most likely to improve the model’s performance if labeled. This selective process not only speeds up the training time but also cuts down on the resources required for data labeling.
The Cost-Effectiveness of Active Learning
Data labeling can be prohibitively expensive, especially for large datasets commonly used in AI applications. It is estimated that data preparation accounts for about 80% of the work involved in machine learning projects, with a significant portion of this cost stemming from manual data labeling (Monarch, 2021). Active learning mitigates these costs by reducing the number of data points that need to be labeled. Studies have shown that active learning can reduce data labeling costs by up to 50% while maintaining or even improving the accuracy of machine learning models (Johnson, 2022).
Addressing Common Concerns
Despite its benefits, some may worry about the initial setup and complexity of integrating active learning into existing ML workflows. While it is true that active learning requires upfront investment in terms of setup and integration, the long-term savings and efficiency gains make it a worthwhile endeavor. Moreover, with the advancement of ML frameworks and tools, incorporating active learning has become more accessible than ever.
Another concern is the quality of the selected data points. Skeptics argue that the model might focus on outliers or anomalies, which do not represent the general dataset. However, active learning algorithms are designed to identify data points that are most informative for the model, based on uncertainty or error reduction strategies, thereby ensuring a representative sample.
Real-World Applications and Success Stories
Several leading tech companies have successfully implemented active learning to streamline data labeling and reduce costs. For example, a prominent social media company used active learning to improve the efficiency of its content moderation algorithms, reducing the amount of data that needed to be manually reviewed by 40% (TechCrunch, 2023).
In healthcare, active learning has been used to label medical images, such as X-rays and MRI scans, more efficiently. By focusing only on the most critical images, hospitals have been able to cut down on the time and cost associated with data preparation, while still developing robust diagnostic tools (HealthTech Magazine, 2023).
Conclusion
Active learning represents a significant leap forward in the field of machine learning, offering a practical solution to the challenges of data labeling. By prioritizing the most informative data points, active learning not only streamlines data labeling but also enhances the overall efficiency and effectiveness of machine learning projects. As businesses and researchers continue to seek cost-effective and efficient solutions, active learning stands out as a promising approach that can reduce data labeling costs by up to 50%, thereby accelerating the pace of AI innovation and adoption.
In conclusion, embracing active learning is not just about cutting costs—it’s about making smarter use of data to drive faster, more accurate decision-making in machine learning. As we continue to navigate the complexities of AI and data science, active learning will undoubtedly play a crucial role in shaping the future of technology.
By integrating active learning strategies, organizations can achieve more with less, ensuring that their machine learning initiatives are not only cost-effective but also positioned for success in the competitive landscape of AI development.